A similar version of this was presented at the UConn Sports Analytics Symposium and is published in the Wharton Sports Analytics Journal. I wrote this article in June for a more general audience than my usual readership, so it has a slightly different tone.
“The ref is biased!” hollers an angry fan at every sporting event. From subjectively judged sports like figure skating to seemingly clear-cut sports like swimming, every sport has had some officiating scandal. These referee errors not only disappoint players, coaches, and fans—nobody likes unfairness—but can also have a large economic impact [1]. For example, one referee’s unjustified yellow card to a player on the soccer team Bayern Munich cost the team over 20 million euros in potential earnings from winning. Consequently, it is important to understand what causes these errors and why they happen.
A recent controversy in fencing
Lately, fencing, the sport of sword fighting, has been moored with controversy [2]. At a recent United States Olympic qualifying tournament, referees were caught colluding with an Olympic hopeful’s coach, helping her win a bout and eventually advance to the semifinals. Additionally, strong evidence was found that some officials were favoring certain fencers at prestigious international tournaments. This evidence was so damning that USA Fencing, the American national governing body, asked the International Fencing Federation to no longer assign those referees to American fencers’ bouts.
It’s easy for officials to cheat in fencing because of how subjective the rules are. In two of the three fencing weapons, foil and saber, there is a subjective system called “right-of-way.” This system gives referees nearly full discretion in awarding the point when two fencers land hits simultaneously, which happens quite frequently. Epee, the third fencing weapon, doesn’t have right-of-way, so both fencers can be awarded points on simultaneous touches. Still, epee remains subjective because referees can annul touches and issue penalties for bodily contact.
The intimate fencing community could have problems
Members of the fencing community, even throughout the vast United States, are well connected. Unlike mainstream sports like baseball and soccer where most athletes play in local sporting leagues, fencing requires a substantial amount of travel, even for amateur athletes. USA Fencing, the national governing body for fencing, incentivizes this traveling. For athletes to qualify for the prestigious National Championships and Junior Olympics, they must accumulate enough “regional points” by placing well at regional tournaments. Fencers are assigned to one of six regions based on their location of residence and can only earn regional points at tournaments in their own region. These regions are far-reaching, covering multiple states, and there are a limited number of regional tournaments. As a result, fencers must travel all over their region to qualify for the championship events.
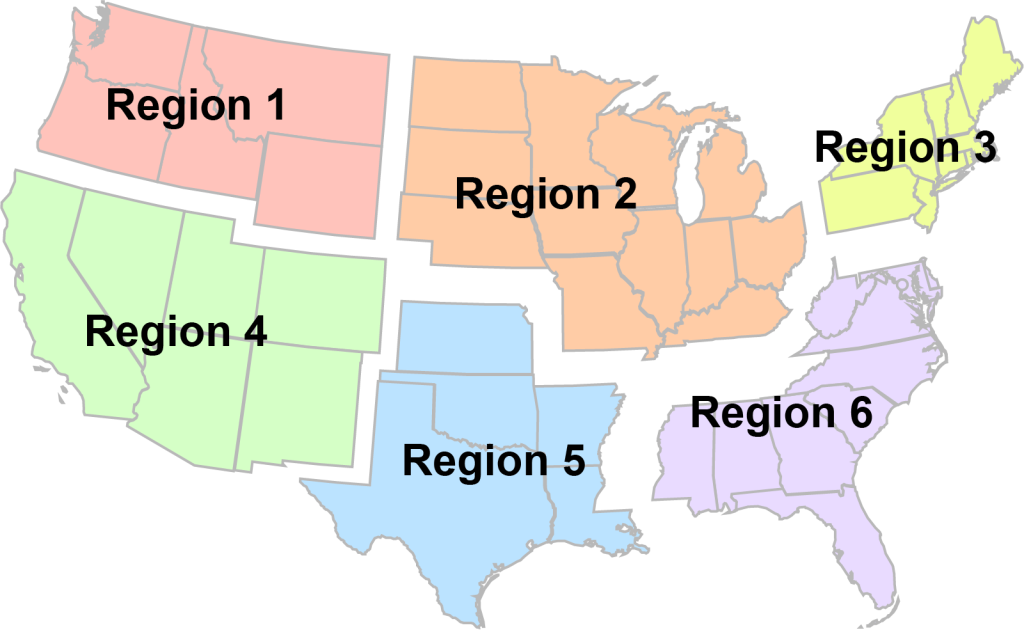
From constant travel and mingling at regional tournaments, referees, coaches, and fencers build relationships and familiarity. This made me wonder if this familiarity could cause referee bias. It has in other sports: both soccer referees and baseball umpires have been shown to favor familiar players; players who lived in close geographic proximity [3], who they had officiated before [3], or who they interacted with frequently during a game [4]. USA Fencing even acknowledges the idea that there could be a regional or familiarity bias: at national tournaments referees are prohibited from officiating fencers from their own division, a small part of a region, about the size of a large metropolitan area or a small state.
The natural experiment
It is convenient to measure regional bias in fencing. At national tournaments, there are two stages: pools, and direct elimination. In the pools round, which determines the seeding going into the elimination round, fencers are placed into pools—groups of 6-7 other competitors—and fence a round-robin where each competitor fences each of the other competitors in the pool exactly once. Importantly, referees are randomly assigned to officiate these pools. This is akin to a randomized controlled trial, an experimental method often used in clinical studies to measure the effect of a drug or treatment. In fencing, the referee randomly being from the same region as a fencer is the same as the drug being randomly assigned to the study participant. This “natural experiment” allows us to determine if there is an effect from the referee being from the same region as the fencer, so we can assess if there is a regional or familiarity bias.
My first instinct was to simply compare the bouts where the referee and fencer were from the same region to bouts where the referee and fencer were not from the same region. Then I could see whether fencers had a higher chance of winning when the referee was from the same region.
Foil | # of wins | # of losses | % chance of victory |
Referee from the same region | 395 | 394 | 50% |
Referee from a different region | 1882 | 1907 | 50% |
Epee | # of wins | # of losses | % chance of victory |
Referee from the same region | 369 | 343 | 52% |
Referee from a different region | 1684 | 1756 | 49% |
Saber | # of wins | # of losses | % chance of victory |
Referee from the same region | 520 | 481 | 52% |
Referee from a different region | 1996 | 2043 | 49% |
At first, it looks like referees are biased towards fencers from their own region! In epee and saber, fencers have a 3% higher chance of victory when the referee is from their region.
The hidden back door path
But wait, there’s a catch! Even though referee assignment is random, a referee being from the same region as a fencer depends on the fencer’s own region. This is problematic because of variability in regional strength. For instance, saber fencers from the east coast (Region 3) are stronger, winning 53% of their bouts as opposed to the southeast’s (Region 6) 48%. Additionally, there are over 2 times more Region 3 referees and fencers in the sample than Region 6. Consequently, of the bouts where the referee and fencer are from the same region, a higher proportion of them are from Region 3, a generally stronger region.
If we were to model this idea as a directed acyclic graph (DAG), a method for representing causal relationships, it would look something like this:
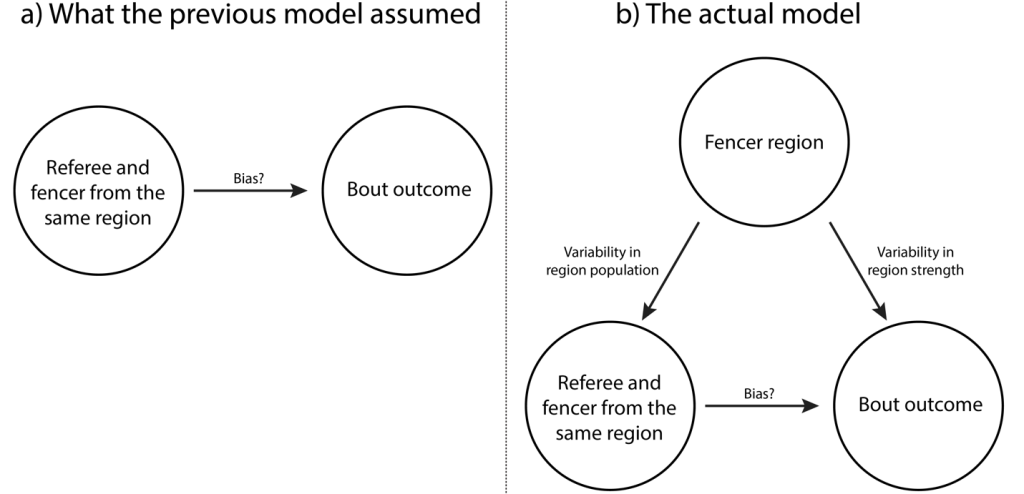
In the context of a DAG, the confounding in Figure 2b is called a back door path, since it is another way of getting from the treatment (referee and fencer being from the same region) to the outcome. Not all is lost though. We can solve this by looking at the causal effect of the referee and fencer being from the same region on bout outcome while holding fencer region fixed. This effectively reverts us back to the situation described in Figure 2a, since fencer region is unchanging and no longer able to influence either of the variables.
One technique we can employ to hold fencer region fixed is multiple logistic regression, a method to isolate the effect of multiple different factors on a binary outcome. By using the referee and fencer being from the same region and the fencer’s region as our variables, the regression model can measure how assigning a referee from the same region as a fencer affects how likely the fencer is to win, in isolation of the effect of the fencer’s region.
Weapon | Foil | Epee | Saber |
Effect of referee and fencer being from the same region on log-odds ratio of bout outcome (95% CIconfidence interval a range of values calculated from sample data that is likely to contain the true population parameter, providing a measure of the uncertainty or precision of an estimate) | -0.017 (-0.173, 0.139) | 0.079 (-0.088, 0.245) | 0.007 (-0.140, 0.153) |
You might notice that the table is measuring the “log-odds ratio,” rather than the increase of chance in winning. Logistic regression uses the natural log of the odds ratio, ln(P(win)/P(loss)), which has special properties that make it easier to estimate the effect from other variables. However, the log-odds ratio is functionally very similar to the chance of victory: as you increase the chance of winning, the log-odds ratio also increases. A log-odds ratio of 0 means that there is no effect.
Since zero is within the confidence intervalconfidence interval a range of values calculated from sample data that is likely to contain the true population parameter, providing a measure of the uncertainty or precision of an estimate for all three weapons, the results are no longer statistically significantstatistically significant a result in statistical testing that provides enough evidence to reject the null hypothesis, suggesting that an observed effect is likely not due to chance alone, meaning that there is no evidence of causal effect of the referee and fencer being from the same region on the bout outcome in Division I pool bouts.
Takeaways
Is there systematic regional bias in fencing? We can never be 100% confident in a statistical analysis; we could have missed an important confounder or found a strange result due to chance. However, statistics are still an excellent objective method for understanding the subjective world around us. Based on our results, there is no evidence of referee bias. With a sample sizesample size the number of individual observations or data points collected from a population for use in statistical analysis or experimentation of over 4,000 bouts for each weapon, even an incredibly small bias would be statistically significant, which makes this nonsignificantnonsignificant a result in statistical testing that does not provide enough evidence (often a p-value < 0.05) to reject the null hypothesis, suggesting that any observed effect may be due to chance result especially surprising [5]. Why was there evidence of familiarity bias in soccer in baseball, but no evidence in fencing, where the referee holds so much powerpower the probability that a study will correctly reject the null hypothesis when it is false, indicating the sensitivity of a test to detect an effect if it truly exists ?
What we can take away from this is that it’s important to control for confounding variables in statistics—after controlling for fencer region we found that there is probably no referee bias. This phenomenon is called Simpson’s paradox, which is when trends in specific groups can change or reverse when combined. Simpson’s paradox is not only relevant to analyzing referee behavior but can also cause problems with real-life data. In one famous example [6], researchers concluded that UC Berkeley was biased against women, admitting 46% of male and 35% of female applicants. Analyzing each department, they found that women were generally applying to more competitive subjects, and within many departments, they had higher admission rates than men!
Just like fencing, where you precisely manipulate your opponent until you create the perfect opportunity to hit, finding a conclusion in statistics requires diligence and precision to avoid falling prey to confounders.
References
[1] Albanese A, Baert S, Verstraeten O. Twelve eyes see more than eight. referee Bias and the introduction of additional assistant referees in soccer. PLOS ONE. 2020 Feb 26;15(2). doi:10.1371/journal.pone.0227758
[2] Longman J. Fencing rattled by suspensions and accusations ahead of Olympics [Internet]. The New York Times; 2024 [cited 2024 May 23]. Available from: https://www.nytimes.com/2024/05/09/world/europe/fencing-olympics-turmoil.html
[3] Hlasny V, Kolaric S. Catch me if you can. Journal of Sports Economics. 2015 Jun 9;18(6):560–91. doi:10.1177/1527002515588955
[4] Mills BM. Social pressure at the plate: Inequality aversion, status, and mere exposure. Managerial and Decision Economics. 2013 Jul 5;35(6):387–403. doi:10.1002/mde.2630
[5] Abadie A. Statistical nonsignificancenonsignificant a result in statistical testing that does not provide enough evidence (often a p-value < 0.05) to reject the null hypothesis, suggesting that any observed effect may be due to chance in empirical economics. American Economic Review: Insights. 2020 Jun 1;2(2):193–208. doi:10.1257/aeri.20190252
[6] Bickel PJ, Hammel EA, O’Connell JW. Sex bias in graduate admissions: Data from Berkeley. Science. 1975 Feb 7;187(4175):398–404. doi:10.1126/science.187.4175.398